We wouldn’t be serious about AI if we didn’t jump on the DeepSeek bandwagon.
It’s remarkable how it shook up the AI world. Not because it was the smartest model. Not because it was breaking records on academic leaderboards.
Because it was cheaper.
The DeepSeek moment signals a shift in AI. For years, the industry operated under a maximalist philosophy. Starting with GPT-1, each successive generation was orders of magnitude bigger (and more expensive) than the last. The logic became self-reinforcing: a model was considered better because it was bigger, and it had to be bigger because that’s what made it better.
To prove this was true, we relied on tests—esoteric benchmarks understood only by AI researchers, along with more familiar but equally dubious measures, like the SAT and the bar exam, which GPT-4 passed (or did it?).
But did any of this make AI meaningfully better for users? Arguably not. That’s not to say AI hasn’t improved since OpenAI launched ChatGPT in 2022—it has. But the real progress didn’t come from pushing intelligence to its theoretical limits through ever-bigger models. It came from making AI faster, cheaper, and more accessible.
If I’d asked people what they wanted…
…they’d have said a faster horse, said Henry Ford (or did he?).
Depending on who you ask, that maxim is either an indictment of market research—a call for visionary inventors to ignore what consumers say to build what they need—or the opposite: a lesson in deep empathy, showing that true innovation comes from understanding the intent behind what people ask for, not just taking their words at face value.
Both interpretations, though, agree on one thing: faster horses are bad.
But what if they aren’t? What if the real breakthroughs—the ones that reshape industries and change how people live—come not from chasing the grandest vision, but from making things cheaper, easier, and well, faster?
Which AI development do you think made the bigger impact in users’ lives—passing the bar exam or any of the following?
Longer context windows that improve memory in conversations.
Lower inference costs that make AI commercially viable.
Lower latency that makes responses feel instant.
Higher reliability that makes models more dependable in production.
Seamless integration into existing tools.
This isn’t a dismissal of fundamental AI research—pushing the boundaries of what’s possible will always matter. And benchmarks aren’t without use—after all, the remarkable thing about DeepSeek is that it was cheap and performed just as well as proprietary models in industry benchmarks.
But when it comes to real-world impact, progress isn’t measured by leaderboard rankings or viral demos. It’s measured by whether AI makes people’s lives easier in tangible ways. A model that can pass the bar exam doesn’t change much for us. A cheaper model does.
At Collected Company, we call this Market-Aligned Product Development (MAP-D)—the idea that AI should be built not just for intelligence or hype, but for impact. That means two things:
Deep Technical Understanding – Knowing how AI works at a fundamental level to understand what it can do and what it can’t.
Deep Business Understanding – Identifying where AI can actually move the needle for companies.
The biggest leaps don’t come from making something theoretically better; they come from making it practically useful.
This isn’t just a theory—it’s a pattern we’ve seen play out before. And history offers a clear example of what happens when companies mistake technical superiority for real-world impact.
“Better” is an empty ideal. “Faster” is what the people want.
A great example of a leader in market-aligned product development was Ford’s competitor, Alfred Sloan, who headed up GM in the 1920s.
As this HBR blog post points out, GM ate half of Ford’s market share over the course of just 5 years. How? By selling cars “for every purse and purpose.” In other words: products that fit people’s actual needs.
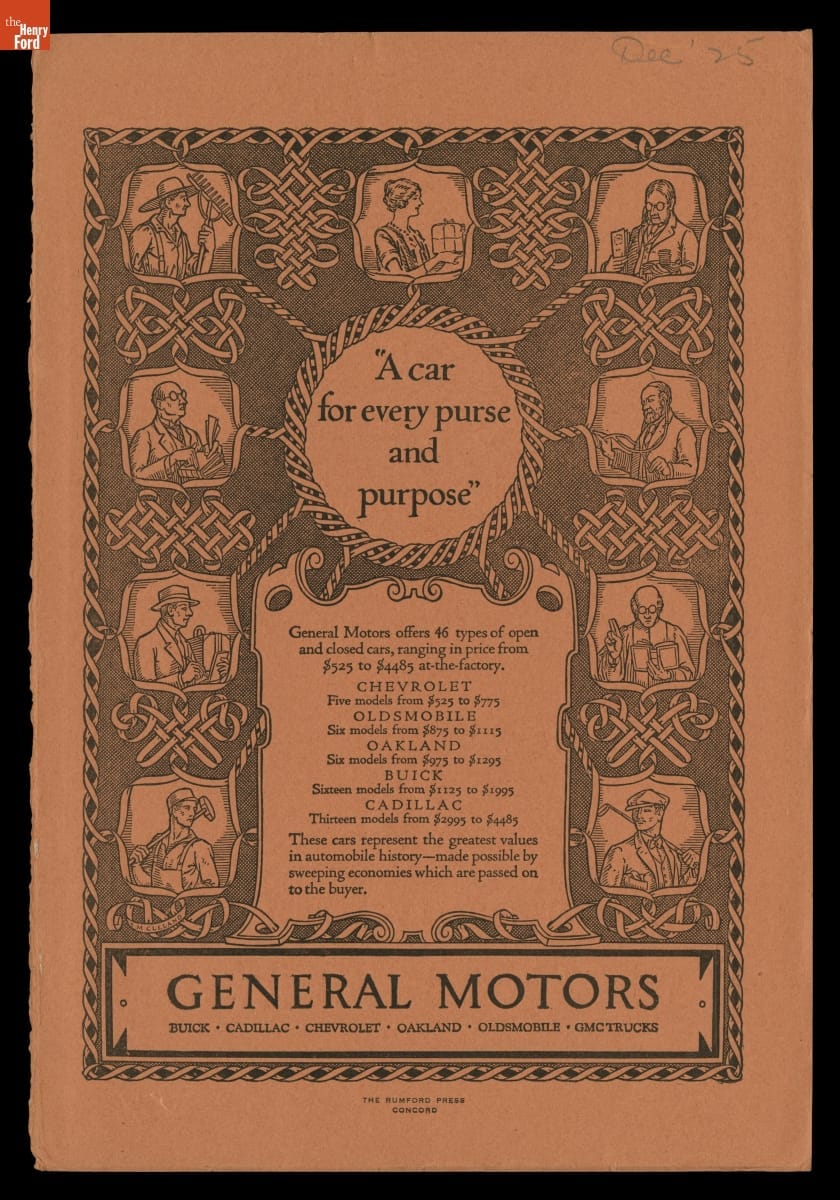
Ford had the better car. The Model T was a marvel of engineering—efficient to produce, technically advanced, and built to last. But better is slippery. GM, on the other hand, made cars that were more practical, more customizable, and more accessible to different kinds of buyers. That’s what actually mattered.
The AI industry has the same problem. It chases better—models that score higher on benchmarks, pack in more parameters, and showcase flashier capabilities. But better is an abstraction, defined and redefined by whatever metric happens to be in vogue.
Faster, though? Faster is real. Faster is felt. It’s the difference between an AI tool that seamlessly integrates into a workflow and one that slows it down. Between something people rely on and something they abandon.
The biggest impact in AI won’t come from pushing intelligence to its theoretical limits. It’ll come from making AI work the way people actually need it to. And most of the time, that means not just making it better, but making it faster, cheaper, and easier to use.