Most essays like this begin with a stat about AI spreading through the marketing industry like wildfire. Everywhere—in surveys from the MIT Technology Review, Deloitte, Salesforce, BCG, and Statista—marketers are “exploring,” “adopting,” and “utilizing” the “critical” technology that is AI.
These studies are silly. Asking someone whether they’re using a tool is not nearly as useful as asking whether that tool is any good—and, most importantly, why.
That’s where this essay comes in. We’re going to walk through the following marketing use cases and qualitatively evaluate how good AI is at each:
Generating imagery
Naming products and features
Writing marketing copy
Validating marketing hypotheses
Analyzing data
Streamlining marketing operations
Importantly, we’ll also dig into why AI fares well or poorly in these areas, focusing on the technical underpinnings that make it so.
We won’t be teaching you prompt engineering or telling you which specific tools are best for each task; rather, we’ll give you a framework for understanding why the technology shines in some contexts and flounders in others.
Methodology
Before we start, three notes on methodology:
A qualitative lens: There isn’t a simple metric for “good marketing,” so we rely on our experience—both authors started their tech careers as product marketing managers. To evaluate AI’s performance in each use case, we ask, “If an intern produced this work, would we think it’s any good?”
Focus on models, not prompts: A skilled prompt engineer will, without question, get better results than what we provide below as examples. However, the underlying model constraints remain. Our examples highlight those baked-in limits, regardless of how expertly you phrase the request.
Tools keep evolving: What’s true in December 2024 might be outdated six months from now. But by focusing on how these models fundamentally function, we hope to give you a clear lens for evaluating any new tools that come along.
Generating Imagery
How it does
Hit-or-miss. Performance really depends on what you’re trying to generate. AI tools are good at softer, organic-looking visuals, like people and animals.
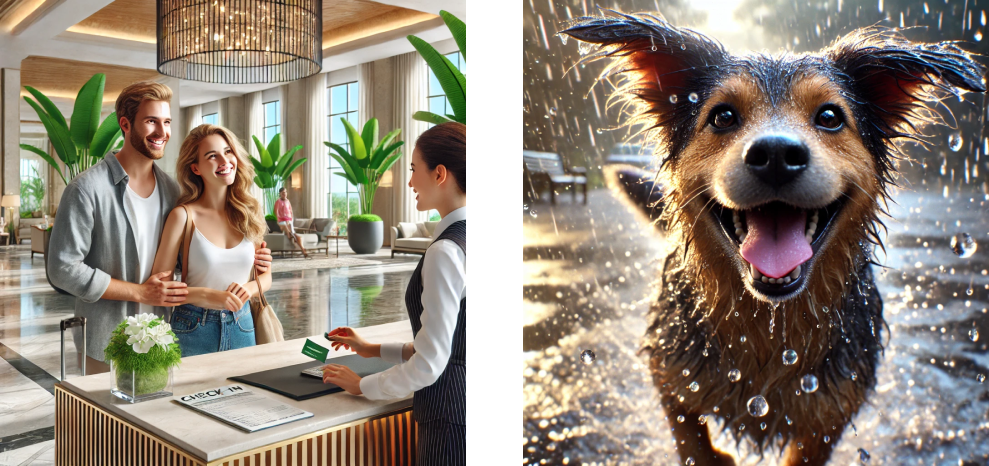
But they struggle with crisp, clean images with sharp contrasts and straight lines. This makes them bad at things like UI and logos.
In the logo below, the plane is asymmetrical, several lines are uneven, and there’s a smudge near the bottom edge—all of which detract from the precision needed for a polished logo.
And the UI is, well, bad.
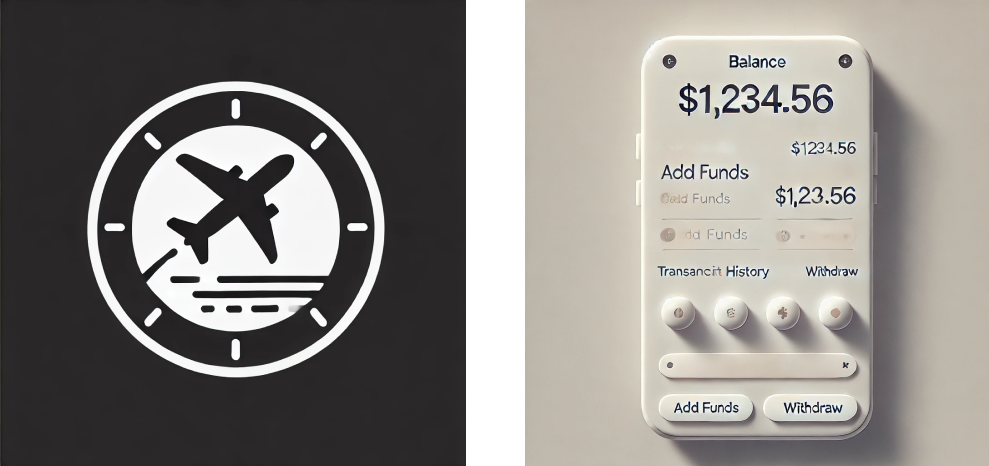
It also makes them bad at product imagery. While the plane in the example below is convincing, if I were a marketer at Boeing, I’d worry about whether details like the size of the wings or the position of the engines actually match a Boeing plane.
The Technical “Why”
Data matters: Most image generation models train on massive datasets of organic, everyday images. That’s great for generating “soft” visual content—natural lighting, fuzzy edges, and broad shapes—but less helpful for the vector-like precision or brand consistency needed for product imagery or logos.
Model architecture: Many popular tools use diffusion models. These algorithms start with noise and iteratively refine the image. This means they excel at approximating complex, “messy” images but struggle with structured or rigid designs like infographics, product mockups, or logos—anything needing tight, consistent lines and proportions.
Tools to Try
DALL-E in ChatGPT: The easiest way to generate images.
Adobe Photoshop (Generative Fill): Lets you easily add or remove elements in an image.
Imagen 2: Has been tuned for background swaps. (Note: As of December 2024, Imagen 2 is only available via API, so implementation will require a technical team. We can help.)
Naming
How it does
Very badly. When it comes to naming, AI models usually return bland suggestions that are not ownable (“Stride” for a shoe brand) or awkwardly mashed-up hybrids (“UrbanAthlete” or “FusionStep”).
While this might be okay for initial brainstorming, it rarely yields the kind of fresh, memorable brand names that truly stand out.
The technical “why”
Tokenization limits creativity. Many names are wholly invented words (“Xerox,” “Expedia”) or altered versions of existing words (“Verizon”). Language models are technically incompatible with this type of thinking, since they work by predicting sequences of “tokens,” which are typically chunks of words (e.g., “strid” + “e”) rather than single letters or syllables. Because of this, the model cannot “invent” words at a granular, character-by-character level—an “X” followed by an “E” followed by an “R” followed by an “O,” etc. Instead, it rearranges existing pieces of language. That’s where we get the recycled feel of AI-generated names.
Tools to try
Don’t expect magic—but if you want to see for yourself, you can test out ChatGPT, Gemini, or Claude.
Writing marketing copy
How it does
It depends. When tasked with writing marketing copy, AI models typically produce text that’s clear and coherent—but also bland and predictable.
This can be helpful for expressing something plainly, or adapting an existing message—shortening a paragraph, or modularly changing the highlighted features based on the audience.
What AI can’t deliver, however, is the kind of unique insight or compelling “hook” that makes your product stand out. Marketing copy isn’t just about clear writing—it’s about telling a story that highlights a product’s unique selling proposition. AI is optimized for what’s statistically common; “unique” is not its strong suit.
The copy below, for instance, could apply to any maker of athletic shoes.
The technical “why”
Generative AI models are fundamentally designed to generate output that’s “likely,” not “original.” Language models predict text by choosing the most probable next word at each step. This probability-driven method means the final output avoids the unconventional phrases that make for unforgettable marketing. Consider taglines like “Think different” (Apple) or “Impossible is nothing” (Adidas). They break standard grammar or common phrasing to grab attention. Because AI picks what’s statistically likely, it usually steers clear of these bold, memorable choices.
Tools to try
Language models like ChatGPT, Gemini, or Claude.
Validating marketing hypotheses
How it does
Surprisingly good—for now. Recent research from HBS and Haas/Columbia/University of Alberta suggests that LLMs can mimic human survey responses with striking accuracy—up to 75% similarity. In theory, this means marketers might skip sending consumer surveys and simply ask an AI to review and validate concepts.
The technical “why”
At their core, language models choose the most likely next words based on massive datasets and continual human feedback. In other words, they’ve learned to be “mid”—to converge around the average human response. So, if you ask these models what people think of a new product idea or marketing angle, they can provide a reasonable stand-in for an actual focus group.
However, there are a few important caveats:
Layered representation problem. Surveys are already a representation of real-world opinion. Using an AI (another layer of representation) on top of that can feel like you’re stacking abstractions—fine in theory, but it may not always capture genuine consumer sentiment.
Panel selection still matters. While these studies show LLMs can “impersonate” certain demographics, niche groups are harder to emulate. If the model hasn’t seen enough data from a specific subset of people, its responses are less reliable.
Changing data mix. As training data evolves—especially with more synthetic inputs—and as models shift toward machine-based fine-tuning methods, LLMs may drift further from the human “median.” Today’s near-accurate results could become less trustworthy tomorrow.
Tools to try
If you’re curious, you can experiment with ChatGPT, Gemini, or Claude for a quick “pseudo focus group.” Just remember you’re dealing with a statistical approximation, not a direct pipeline to collective human consciousness.
Data analysis
How it does
Pretty darn good. AI has powered data analysis for quite some time, though usually through quantitative methods like regression or clustering. Even so-called “qualitative” analysis still relied on quantitative methods, ignoring the actual semantic content of things like product reviews or interview transcripts.
But with LLMs, you can feed large sets of qualitative data into a model and get back meaningful insights—like recurring themes in user feedback or notable quotes from focus groups. Instead of simply grouping or ranking data objects, LLMs can actually interpret them, generating high-level summaries that capture the essence of what people are saying.
The technical “why”
Well represented in the data. A lot of content on the internet follows the same structure: data, then conclusion—think scientific papers, well-reasoned opinion pieces, or even Reddit threads (on a good day). LLMs have essentially “learned” this structure from massive training sets.
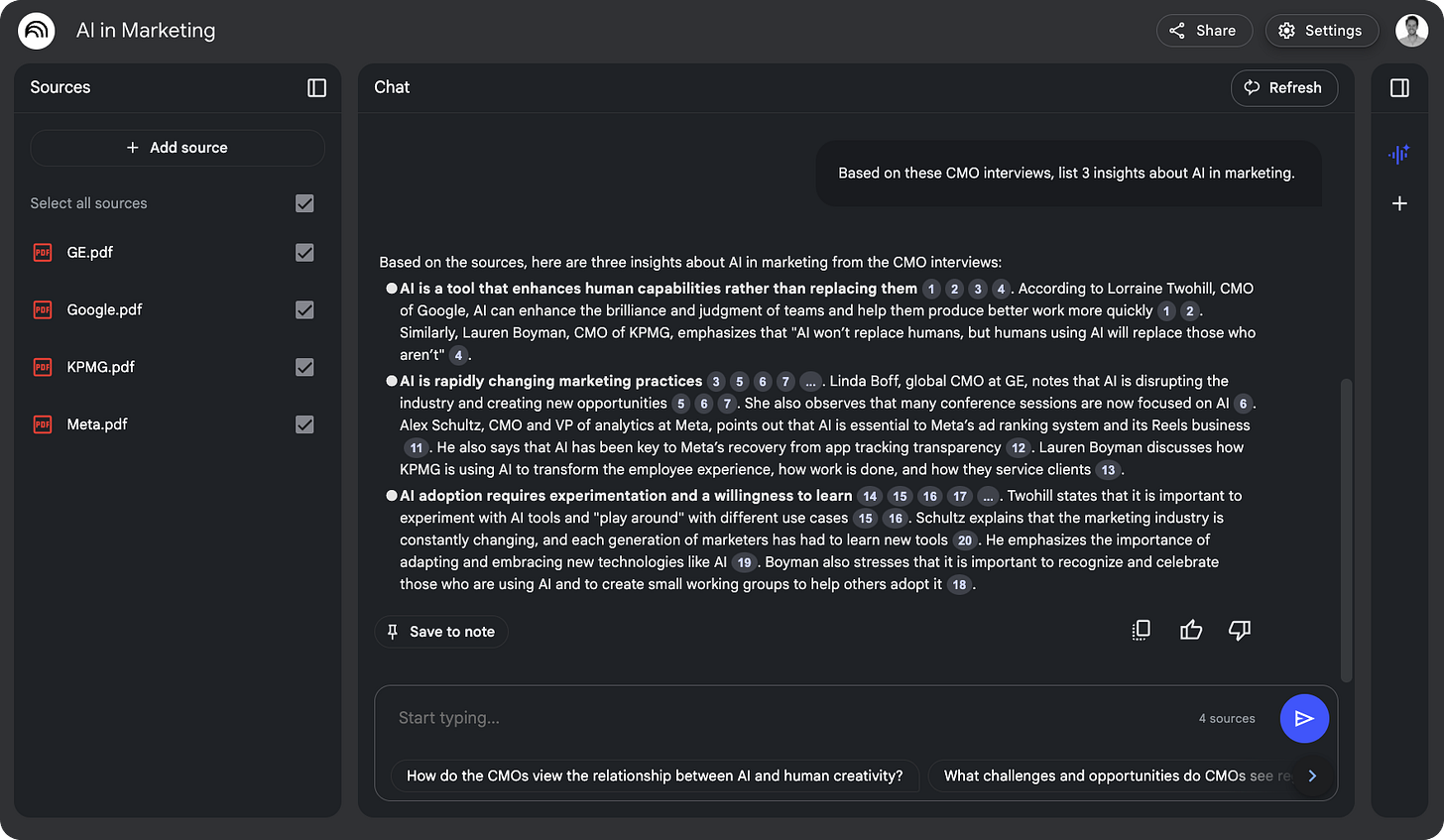
Tools to try
NotebookLM and Perplexity. These platforms let you upload, query, and summarize your data, helping you move beyond raw numbers into more nuanced, qualitative insights.
Marketing operations
How it does
Good—though we’re using “operations” as a broad umbrella to mean all the small tasks that keep workflows humming. For instance:
Getting help writing a clearer, more concise email
Using meeting transcripts to generate executive updates
Using AI to annotate assets so they’re easier to find later
None of these tasks are especially glamorous, but they can have a noticeable impact on both productivity and output quality when aggregated over time.
The technical “why”
Large AI models excel at tasks that mirror how they’re trained: in discrete, narrowly defined steps with clear feedback. Breaking a real-world job into smaller pieces—like “extract themes from this data” or “polish this paragraph”—mimics that training process, making it easier for the model to perform well.
As a rule of thumb, if you can split a process into smaller, data-heavy components, an LLM can probably handle one or two of those steps effectively—whether that’s summarizing transcripts, labeling images, or writing short blurbs.
Final Thoughts
Marketers should understand why AI works. These models aren’t magic; they excel at tasks that align with their technical underpinnings and struggle when we ask them to invent something genuinely new or nail the tiniest details.
Keep that in mind, and you’ll know exactly where AI can supercharge your workflows.